- Combining Simulation Models and Big Data Analytics for ATM Performance Analysis
- Consortium: Nommon (coordinator), CRIDA, Fraunhofer Society, University of Piraeus Research Centre, and Technical University of Catalonia.
- 2021 – 2022
- www.simbad-h2020.eu
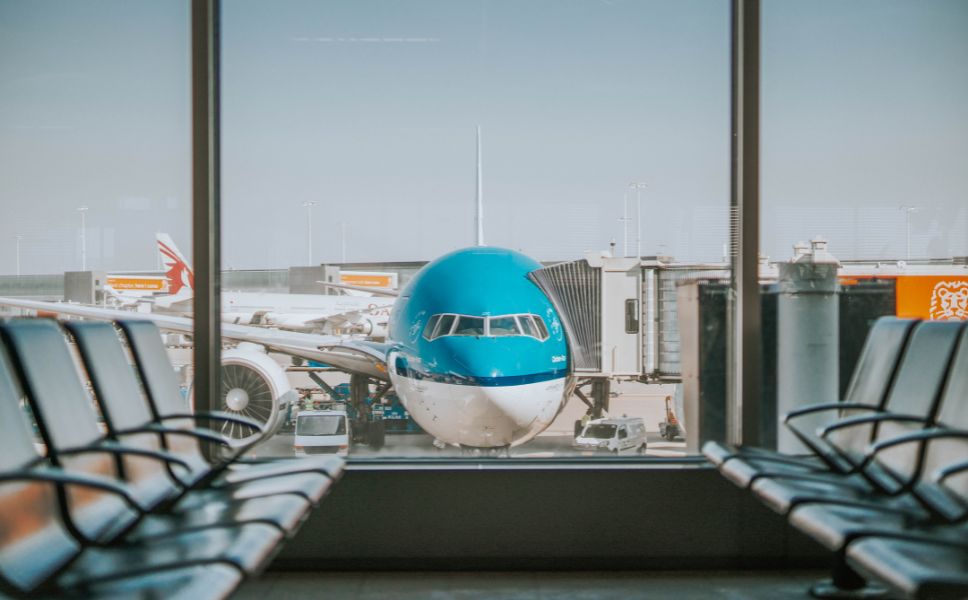
The SIMBAD project
The development of performance modelling methodologies able to translate new ATM concepts and technologies into their impact on high-level, system-wide KPIs has been a long-time objective of the ATM research community. Bottom-up, microsimulation models are often the only feasible approach to address this problem in a reliable manner. However, the practical application of large-scale simulation models to strategic ATM performance assessment is often hindered by their computational complexity. The SIMBAD project developed an ATM Performance Modelling Framework aimed at enhancing the capabilities of large-scale ATM microsimulation models to effectively support performance evaluation at network level. The framework enables the comprehensive exploration and understanding of the performance impact of ATM concepts and solutions under different traffic conditions.
Goals
The goal of SIMBAD was to develop and evaluate a set of machine learning approaches aimed at providing state-of-the-art ATM microsimulation models with the level of reliability, tractability and interpretability required to effectively support performance evaluation at ECAC level. The specific objectives of the project were the following:
- Explore the use of machine learning techniques to estimate hidden variables from historical air traffic data, with a particular focus on airspace users’ preferences and behaviour. This aimed to enable a more robust calibration of air traffic microsimulation models.
- Develop new machine learning algorithms for classifying traffic patterns that enable the selection of a representative set of simulation scenarios, allowing a comprehensive assessment of new ATM concepts and solutions.
- Investigate the use of active learning metamodelling to facilitate a more efficient exploration of the input-output space of complex simulation models through the development of more parsimonious performance metamodels, i.e., analytical input/output functions that approximate the results of a more complex function defined by the microsimulation models.
- Demonstrate and evaluate the newly developed methods and tools through a set of case studies in which the proposed techniques will be integrated with existing, state-of-the-art ATM simulation tools and used to analyse a variety of ATM performance problems.
Nommon’s role
Nommon coordinated the SIMBAD project and led the work related to the development of metamodelling and active learning techniques for ATM microsimulation tools. Nommon developed metamodels for two state-of-the-art ATM microsimulation tools: DYNAMO and RNEST. These metamodels are able to approximate the performance impact of different ATM solutions and concepts, and to identify those regions or scenarios in which a more exhaustive analysis with the microsimulator is required, supporting and complementing the use of these microsimulation models.
Nommon also participated in the design of a cluster-based methodology for the identification of traffic patterns and the selection of representative traffic samples for different geographic and temporal scales, collaborating at the same time in the validation of the methodology.
Results
SIMBAD has delivered an ATM performance modelling framework aimed at enhancing the capabilities of large-scale ATM microsimulation models to effectively support performance evaluation at network level. The framework enables the comprehensive exploration and understanding of the performance impact of ATM concepts and solutions under different traffic conditions. It comprises three main elements:
- A model for the estimation of hidden variables related to airspace user behaviour that are necessary inputs for the ATM microsimulation models, such as cost index and landing weight. The validation exercises conducted by SIMBAD showed how a more accurate estimation of these hidden variables improves trajectory simulation by providing a more realistic representation of airspace users’ behaviour and preferences.
- A methodology to identify representative traffic patterns at different scales for each particular problem under study. This methodology was demonstrated and validated by applying it to two SESAR solutions: Free-Routing and Demand and Capacity Balancing. The validation exercises proved that the SIMBAD approach leads to a much more accurate estimation of certain KPIs (e.g., annual fuel consumed in the ECAC area) than that enabled by a selection of representative traffic days based on expert judgement, while reducing the number of required simulation scenarios.
- A metamodelling framework that enables the approximation of the results of a microsimulation model to facilitate a more efficient exploration of its input-output space. Simulation metamodels of UPC’s DYNAMO trajectory simulation tool and EUROCONTROL’s R-NEST simulation tool were defined and implemented. The predictive performance of the trained metamodels was assessed and analysed, showing their ability to accurately reproduce the behaviour of the microsimulation models while drastically reducing the required computational resources.
The SIMBAD Performance Modelling Framework was demonstrated through a series of case studies in which the different components of the framework (either alone or combined with each other) have been put at work. These demonstration exercises showed how the project results can help overcome some of the main drawbacks of traditional ATM microsimulation models, facilitating a more comprehensive, accurate, and efficient assessment of the performance impact of new ATM solutions/concepts.
Read SIMBAD white paper: Lessons learnt from the SIMBAD project and way forward
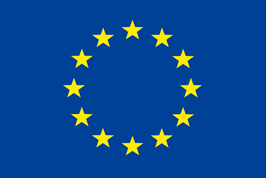
This project received funding from the SESAR Joint Undertaking (SJU) under grant agreement No. 894241. The SJU receives support from the European Union’s Horizon 2020 Research and Innovation Programme and the SJU members other than the Union.