- Interactive Toolset for Understanding Trade-offs in ATM Performance
- Consortium: Nommon (project coordinator), Advanced Logistics Group, Fraunhofer IAIS, Universidad Politécnica de Madrid, and Transport & Mobility Leuven.
- 2016 – 2018
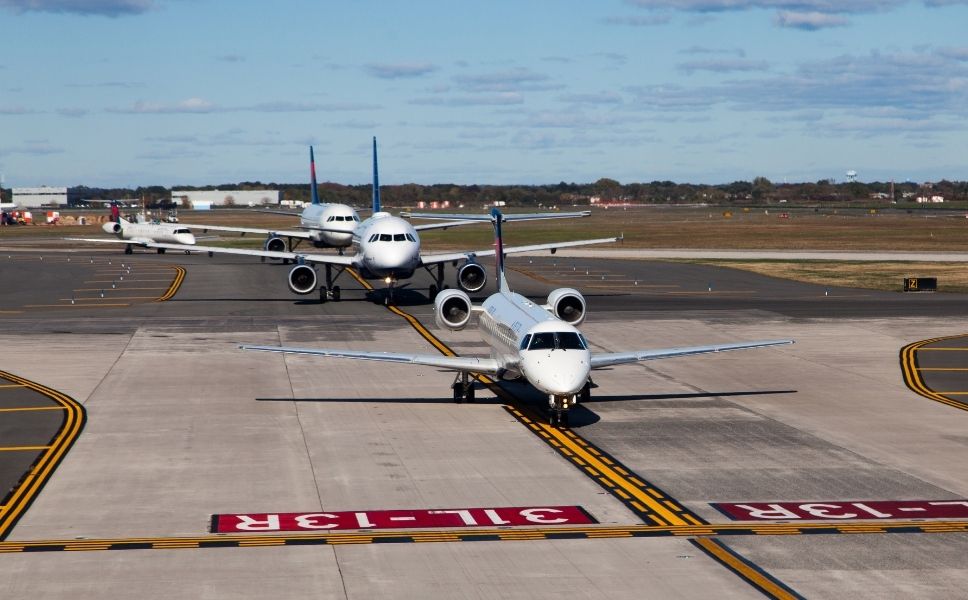
Context
Performance orientation in Air Traffic Management (ATM) is one of the cornerstones of the Single European Sky (SES) and of its technological pillar, SESAR. A performance-based approach is defined by ICAO as one based on defining policy in terms of qualitative performance objectives, making them measurable by defining appropriate indicators, developing the data and methodologies necessary to calculate the indicators, and having the expertise to maintain data quality and assess the link between indicator trends and management actions. However, realising these elements is not an easy task: ATM performance results from the complex interaction of interdependent policies and regulations, stakeholders, technologies and market conditions, and trade-offs arise not only between Key Performance Areas (KPAs), but also between stakeholders, as well as between short-term and long-term objectives. The need for improved performance modelling methodologies, able to grasp the interdependencies between different Key Performance Indicators (KPIs) and enable the assessment of the possible future impacts of a range of policies and technologies, has been acknowledged by the ATM stakeholders and the research community.
The INTUIT project
INTUIT is a SESAR exploratory research project that explored the potential of visual analytics and machine learning to improve our understanding of the tradeoffs between ATM KPAs, and develop new decision support tools for ATM performance monitoring and management. The project was conducted by a consortium composed by Nommon as project coordinator, ALG, Fraunhofer-IAIS, the Technical University of Madrid, and Transport & Mobility Leuven.
The project started by identifying the available data sources on European ATM performance and conducting a detailed assessment of the validity, the quality and the geographical and temporal resolution of each dataset. This work led to the production of a set of Performance Data Factsheets characterising each data source, and a Performance Data Guide that links the different ATM KPAs and KPIs with the sources where such data can be found. Taking this work as a starting point, a combination of literature review and stakeholder consultation led to the definition of a Research Agenda on Data Science and ATM Performance.
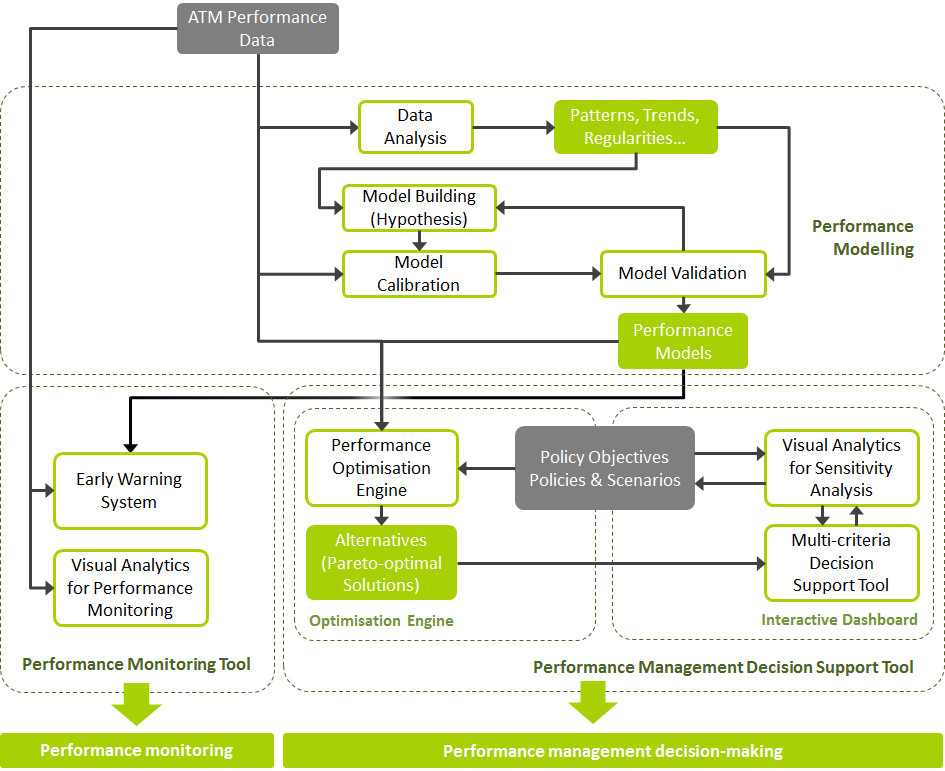
To find more about the project, download INTUIT Position Paper.
Objectives
The specific objectives of the project were the following:
- To conduct a systematic characterisation of the ATM performance datasets available at different spatial and temporal scales and evaluate their potential to inform the development of new indicators and modelling approaches.
- To propose new metrics and indicators providing new angles of analysis of ATM performance.
- To develop a set of visual analytics and machine learning algorithms for the extraction of relevant and understandable patterns from ATM performance data.
- To investigate new data-driven modelling techniques and evaluate their potential to provide new insights about cause-effect relationships between performance drivers and performance indicators.
- To integrate the newly developed analytical and visualisation functionalities into an interactive dashboard supporting multidimensional performance assessment and decision making for both monitoring and management purposes.
Read INTUIT Project Summary to learn about INTUIT case studies and final conclusions.
Public deliverables
- D1.3 Final Project Results Report
- D2.1 Performance Data Inventory and Quality Assessment
- D2.2 Qualitative Analysis of ATM Performance Drivers and Tradeoffs
- D3.1 Visual Analytics Exploration of Performance Data
- D4.1 Performance Metrics and Predictive Models
- D5.1 Performance Monitoring and Management Toolset
- D5.2 Performance Monitoring and Management Toolset Evaluation Report
Scientific papers
- Marcos, R., Cantú Ros, O.G., and Herranz, R., (2017) “Combining Visual Analytics and Machine Learning for Route Choice Prediction: Application to Pre-Tactical Traffic Forecast“, in D. Schaefer (Ed.) Proceedings of the SESAR Innovation Days 2017, EUROCONTROL.
- Andrienko, G., Andrienko, N., Chen, W., Maciejewski, R., and Zhao Y., (2017) “Visual Analytics of Mobility and Transportation: State of the Art and Further Research Directions”, IEEE Transactions on Intelligent Transportation Systems, vol. 18(8), pp.2232-2249.
- Andrienko, G., Andrienko, N., Fuchs, G., and Cordero J.M., (2017) “Clustering Trajectories by Relevant Parts for Air Traffic Analysis”, IEEE Transactions on Visualization and Computer Graphics, vol. PP, no. 99, pp. 1–1.
- Marcos, R., Toribio, D., Alsina, N., Garrigó, L., Andrienko, N., Andrienko, G., Piovano, L., Blondiau, T., and Herranz, R., (2016) “Visual Analytics and Machine Learning for ATM Performance Modelling: Preliminary Findings of the INTUIT Project and Prospects for Future Research“, in D. Schaefer (Ed.) Proceedings of the SESAR Innovation Days 2016, EUROCONTROL.
Presentations
- Marcos, R., (2017) “Combining Visual Analytics and Machine Learning for Route Choice Prediction: Application to Pre-Tactical Traffic Forecast“, presentation at the SESAR Innovation Days 2017, Belgrade, Serbia, November, 2017.
- Marcos, R., (2016) “The INTUIT Project“, poster presented at the SESAR Innovation Days 2016, Delft, The Netherlands, November, 2016.
- Marcos, R., (2016) “Visual Analytics and Machine Learning for ATM Performance Modelling: Preliminary Findings of the INTUIT Project and Prospects for Future Research“, presentation at the SESAR Innovation Days 2016, Delft, The Netherlands, November, 2016.
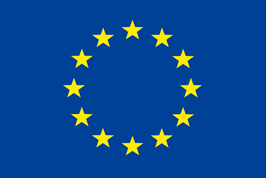
This project received funding from the SESAR JU under grant agreement Nº 699303, under European Union’s Horizon 2020 Research and Innovation Programme.