- A platform for privacy-preserving federated machine learning using blockchain to enable operational Improvements in ATM
- Consortium: SITA eWAS (project coordinator), Nommon, Scaleout Systems, Swiss International Air Lines and EUROCONTROL.
- 2020 – 2022
- aichain-h2020.eu
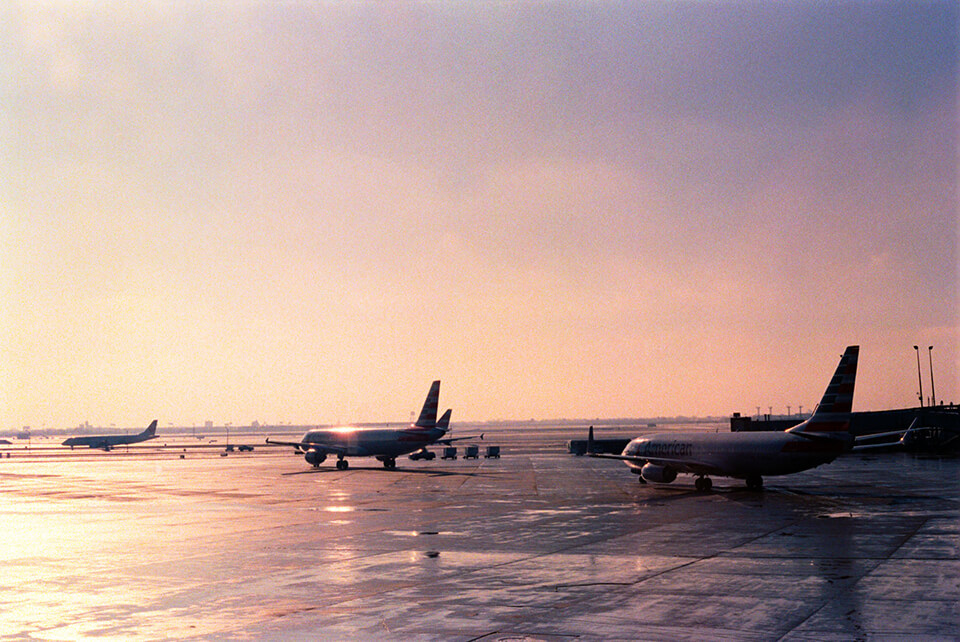
Context
Machine learning (ML) techniques have shown great potential for enhancing prediction and optimization processes in Air Traffic Management (ATM) operations. However, the need of training ML models with historical data poses challenges due to privacy concerns, as stakeholders in ATM are often unwilling to disclose sensitive business information. Additionally, data protection laws impose privacy constraints on data sharing, especially concerning passenger data.
Federated Machine Learning (FedML) emerges as a potential solution to address these privacy issues. FedML allows the training of ML models with the best available data while preserving data privacy. The process involves four iterative steps: 1) the server sends the model to the nodes; 2) the nodes locally train the ML model with their respective data (never leaving the host); 3) each node sends the trained model back to the server; and 4) the server aggregates the models using mathematical functions to create a general model.
Beyond safeguarding data privacy, FedML technologies offer the advantage of accelerating the training of large and complex ML models. This efficiency is attributed to the decentralised and parallelized nature of federated training.
The AICHAIN project
AICHAIN was a research project funded under SESAR 2020 Exploratory Research. The project proposed an innovative Digital Information Management (DIM) concept — the AICHAIN solution — that aimed at enabling the cyber-secured exploitation of large private data sets belonging to different stakeholders and containing valuable information for ATM operations. To overcome the stakeholders’ reluctance to share sensitive data, the exploitation would not be performed by exchanging the data itself, but by articulating an advanced privacy-preserving federated learning architecture in which neither the training data nor the training model need to be exposed. This was possible thanks to the innovative combination of two emerging DIM technologies: FedML and Blockchain technologies.
Goals
The specific objectives of AICHAIN were structured in three areas of research:
- The DIM technological solution: to define the AICHAIN solution’s architecture as a potential SESAR technology enabler for the exploitation of private data value, and to implement a functional small-scale prototype for user validation and operational value experimentation.
- The operational value of the DIM solution: to demonstrate and quantify the operational value of the AICHAIN concept with an ATM use case in the area of A-DCB services.
- The governance & incentives aspects: to develop an incentive mechanism that addresses the motivational aspects of the data owners in order to facilitate the adoption and the effective utilisation of the AICHAIN concept.
Nommon’s role
Nommon played a pivotal role in defining, implementing, and testing case studies to showcase the AICHAIN solution. Specifically, Nommon led the development of a machine learning (ML) model and its integration into the federated architecture for trajectory prediction during the pre-tactical phase. This included scenarios for normal conditions as well as situations with severe weather conditions or constraints related to Demand and Capacity Balancing (DCB). Nommon also led the task of the definition of a governance and incentives mechanisms for the adoption of the AICHAIN solutions.
Results
The research conducted during the AICHAIN project yielded the following outcomes:
- AICHAIN Solution Architecture: the research successfully designed the AICHAIN solution architecture, which is crafted to leverage both private and non-private datasets in a federated manner. The architecture prioritises the preservation of privacy, trust, and scalability.
- AICHAIN Solution Prototype: a prototype of the AICHAIN solution was developed and utilised to showcase the solution through various federated learning experiments. Additionally, the platform underwent cyber-security assessments to ensure robustness.
- Operational Value of Private Data: to demonstrate the practical value of private data, the research showcased its applicability through two Air Traffic Management (ATM) use-cases of high relevance. These operations were notably enhanced with private data sourced from a federated airline.
- Customizable Token Framework: the research introduced a framework of customizable tokens designed to implement rewarding policies. These tokens are strategically aimed at fostering effective collaboration among private data owners participating in federated processes.
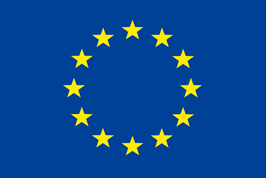
This project received funding from the SESAR Joint Undertaking (SJU) under grant agreement Nº 894162. The SJU receives support from the European Union’s Horizon 2020 Research and Innovation Programme and the SJU members other than the Union.