The nature of mobile phone data
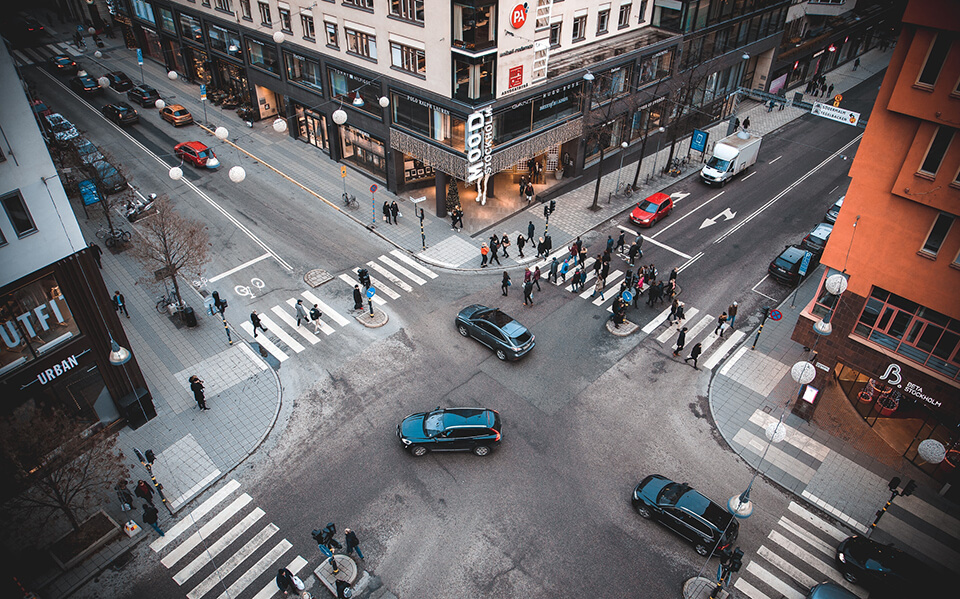
Not all mobile phone trip matrices are born the same [Part 2]
What exactly is mobile phone data? How is it processed to estimate trip matrices? Here we explain five important steps to process mobile phone data in order to generate good quality mobility information.
The simplest piece of information provided by mobile phone data is the CDR (Call Detail Record). This is a data record produced by a Mobile Network Operator (MNO) that documents the details of a phone call or other telecommunications transaction (e.g. text message or data transfer from an email, web search and so on). The CDRs available for processing contain an anonymised (and irreversible) identifier of the user, a time-mark, and the identifier of the antenna and cell used in the connection, plus some additional information not used in mobility studies.
The cell map from the MNO provides the geographical rough coverage of each antenna; this is an area rather than an accurate longitude-latitude spot location (there are some ways to refine locations but they are all process intensive; 5G will make cells even smaller, but these are no relevant to the discussion here). In any case, the size of a 2/3/4G cell is usually smaller than that of most transport model zones. Each CDR then provides an approximate location (small cell in urban areas, larger outside them) and a time stamp. The granularity of these geo-located data points depends on the size of cells and the frequency of phone-antenna interactions.
The general approach to estimate trip matrices (and other mobility indicators) from passive mobile phone data is illustrated in the next figure: Nommon Mobility Analytics Solution.
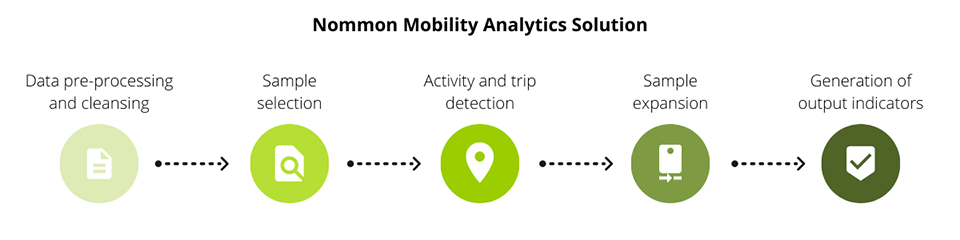
Processing mobile phone data
There are five important steps and each one of them influences the final result.
Data pre-processing and cleansing
The first step is to pre-process and clean the data. The pre-processing usually involves selecting the useful data points and organising them for efficient processing. Mobile phone data is not error free and must be cleansed before further analysis. Mobility data providers, like our Nommon company, have developed their own filters and algorithms to detect errors and eliminate registers that are considered to be unreliable or faulty. This happens more often in early applications with an MNO (sometimes errors in the location of antennas) but remains true over time as, for example, the cell map must be regularly updated. The quality of this first step affects, of course, all further processing of the data.
Sample selection
The second step is to select a useful sample as not all phones generate useful data (for example, there are SIMs that do not move at all as part of the Internet of Things). The potential effective sample comprises those users generating useful information about their daily activities and trips. The selection of these users is based on a set of criteria related to their mobile phone activity, which shall be enough to determine their mobility patterns with an adequate level of accuracy and reliability. This may introduce some bias to be corrected at a later stage. Again, a sensible selection of this sample and subsequent bias correction affects the quality of the resulting matrix.
Activity and trip detection
The next step is the identification of the sequence of activities undertaken by the user. This starts from inferring the place of residence based on the user’s longitudinal behavioural patterns during several days/weeks. This location is later on used to select the sample for a project and to expand such sample to the total population under study.
An “activity” is defined as an interaction or set of interactions with the environment that takes place in the same location and motivates an individual to move there. A “trip” is defined as a sequence of one or more displacements (“stages” or “legs”) between two consecutive activities. This way, a trip has a main purpose identified by the activity at the destination. The algorithms developed by Nommon combine different criteria based on stay times, itineraries and longitudinal behavioural patterns to identify activities, trips, intermediate stops subordinate to the trip (e.g. a stop to change to another transport mode in a multimodal trip, etc.) and the tours connecting them. The result of this process is the sequence of activities, trips and tours performed by each user in the sample and for the period of study. The parameters used to define them are usually refined over time and may be project dependent; they will also influence the final trip matrix.
Sample expansion
The next step is the expansion of the sample to the total population while, at the same time, compensating for any bias in the data: the approach used for the upscaling of the sample depends on the characteristics of the study.
In the case of the residents in the country for which the mobile phone records are available, the expansion of the sample involves the use of factors based on the residence location, typically at the level of census tracts. In the case of tourists and visitors from abroad (roamers), other frameworks need to be used (e.g. official statistics on number of tourists and visitors, ports of entry, etc.). Expanding the sample on the basis of the MNO market share is prone to errors and not recommended.
Generation of output indicators
Finally, once the sample has been expanded to the total population, the activity-travel diaries are post-processed to produce the information requested by the client (e.g. origin-destination matrices) with the required level of spatial and temporal aggregation.
The transport modelling market is the most demanding regarding the accuracy of the data generated from mobile phone CDRs. Understanding how this data will be used is therefore of paramount importance in refining the five steps above, in particular the last three. Perhaps, one of the reasons why some clients have found the trip matrices from mobile phone data frustrating is because the specific implementations of steps three to five were too generic rather than specific to transport modelling.
Overall, trip matrices from mobile phone data should be able to provide good quality information about movements in a study area, not just for an average “normal day”, but also for a whole range of days including specific dates. Having said that, mobile phone data still has significant limitations that must be overcome by other means.
Read «The limitations of mobile phone data: Not all mobile phone trip matrices are born the same [Part 3]«