The limitations of mobile phone data
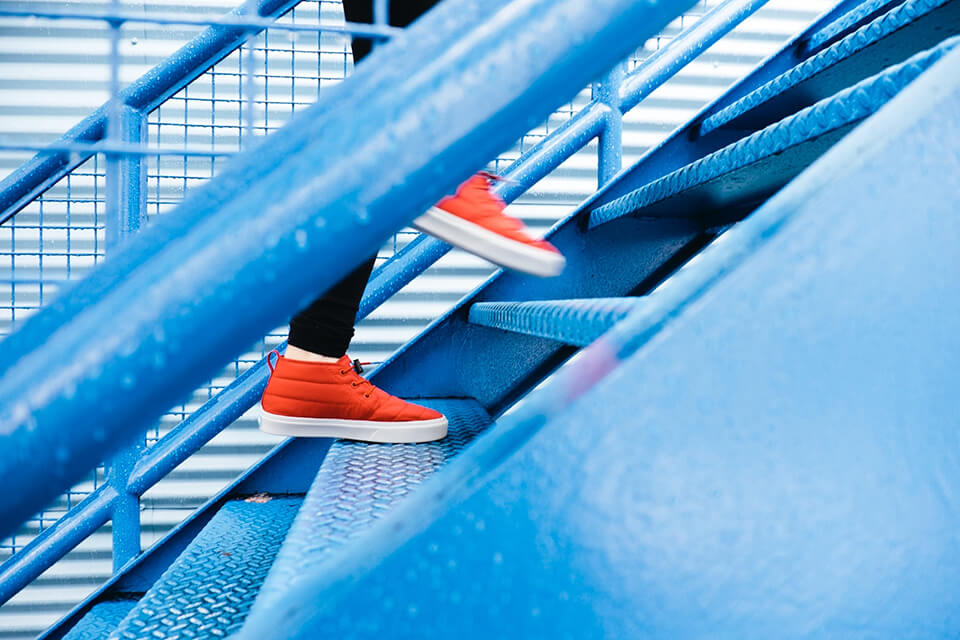
Not all mobile phone trip matrices are born the same [Part 3]
Mobile phone data present some constraints for matrix building. However, some of these limitations can be overcome. In this article we present some of these challenges and how Nommon has found its way to work through them.
Limited granularity
First of all, normally, the granularity of the geo-location is limited by the size of the mobile phone cells, and the granularity of the time-stamps is limited by the frequency of the transactions between mobile phone and antennas (BTS). These two constraints make it impossible to accurately identify the start and end time of any particular trip. Therefore, the estimation of the start and end of a trip and the duration of an activity must be established through probabilistic models.
Another consequence of this limited granularity is an inability to identify short trips that take place within a mobile phone cell. These are mostly walking trips and will become intra-zonal trips in a transport model. It is also important that the algorithms used are able to identify “pseudo-trips”. These apparent movements between adjacent cells may result simply because of the way the mobile network operates: if one antenna becomes overloaded, a call may be transferred to a nearby cell (even if the phone has not moved) creating a pseudo trip. These must be detected (using knowledge about travel and networks) and eliminated from a trip table.
The identification of the Home cell for each user helps associating socio-economic characteristics to the traveller. This may be assisted by some additional information from the contract with the Mobile Network Operator (MNO), for example, gender and type of contract. The identification of the place of work/study is usually achieved by the length and timing of the activity away from home. Recognizing other activities is more difficult as most non-residential cells cover areas of mixed use. Some data analytics companies offer several more journey purposes but in our view this is unlikely to be particularly accurate. The most solid purposes from mobile phone data are Home to Work/Study, Back Home, Other Recurrent and Other Non-recurrent trips. This distinction between recurrent and non-recurrent trips is new and so far not well exploited, given that we know that most trips in a study area are, in effect, non-recurrent. Incidentally, roamers (another user group) provide plenty of non-recurrent trips in an area.
Protection of privacy
The protection of privacy is a central concern of both Mobile Network Operators and Data Analytics companies. The key idea is to provide this protection by design and never offer individual data that could possibly be traced back to an individual. This starts from handling only anonymised information using a unidirectional hash function. Then, only aggregate outputs leave the firewall of the MNO to be provided to the end-user.
There are other ways to achieve this by generating an entirely synthetic population that performs activities and trips modelled on the observations. This is, in essence, what is done when using Household Travel Surveys to produce disaggregate models except that in this case the sample size is at least an order of magnitude bigger and the level of detail includes variability of activities and behaviour on different days and times of the year.
Data fusion
The granularity constraints mentioned above also make it difficult to distinguish car, bus and even bicycle movements in dense urban areas, and the same is true with the precise routes taken by them. Some of these limitations can, and should, be overcome through data fusion.
Good candidates to offer additional information to help this task are:
- Traffic and, when available, person counts.
- The service routes and patterns of public transport.
- Smart Card data, for example, Oyster in London.
- Data from existing Household Travel Surveys.
The availability of one or more of these data sources will certainly help to process and improve the granularity of the trip matrices generated from mobile phone data.
Data science should guide this fusion to make it rigorous and reliable. For example, at Nommon we have combined mobile phone data with traffic counts to provide better trip matrices and routes by vehicle type for toll roads.
One must bear in mind that different data sources have different error distributions, and these must be considered during data fusion. For instance, data from smart cards and bus movements also require cleansing, error and bias correction and sample selection, something we have done when combining it with mobile phone data in urban areas. This is best done when using raw data from different sources.
Overall, data fusion offers the best approach so far to overcome the inherent limitations of mobile phone data. The quality of the resulting trip matrices will depend, of course, on the type of complementary data available and the actual process of data fusion.