Specifying mobile phone trip matrices
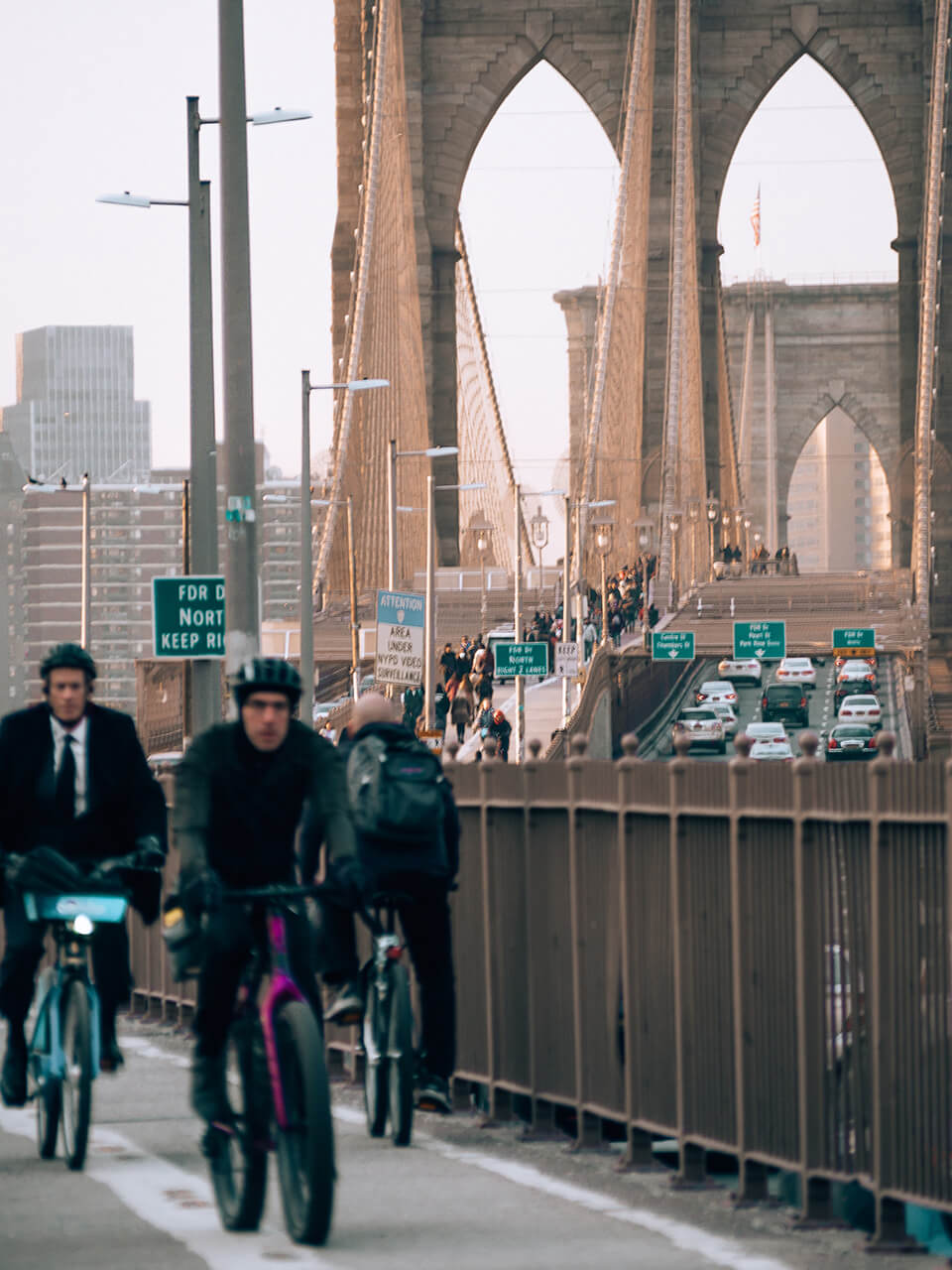
Not all mobile phone trip matrices are born the same [Part 5]
An accurate specification of what is needed is key to obtain usable trip matrices, so here we analyse how to define them in the best possible way.
This section deals with how best to specify the desired trip matrices from mobile phone and other data. In my own experience, with our own mobile phone products and those from other suppliers, a careful specification of what is needed is key to obtain usable trip matrices. All suppliers of this type of trip matrices calculate the cost of delivering them mostly as a function of the granularity requested: the level of detail, the number of zones, days and time periods required by the client. I look at these in turn.
Zoning system
We, at Nommon-Kineo, recommend starting with the zoning system required for transport modelling purposes and discuss this with the data analytics company. An analytics company should be able to comment on the level of detail in case this is too granular for the direct use of mobile data. If the zone details are suitable, the analytics company should be able to adapt its processes to any particular zoning system. This is, in our view, preferable to require the consultant or client to adapt the trip matrices from mobile phone data to the desired zones. This is because the data analytics company is better placed to adapt the sampling, processing and expansion stages to any suitable zoning system rather than post-processing a standard product more coarsely without this knowledge.
Nevertheless, care must be taken to deal with external zones that any model needs to account for all trips. Some Mobile Network Operators find it difficult to provide data for external zones that represent “the rest of the country through a gateway”; this is because the way they select and pre-process the data. In this case, it is better to specify some large zones adjacent to each gateway knowing than some trips from further away will be missing and may have to be adjusted using gateway counts.
Trip and Activity segmentation
As mentioned above, only Home to Work/Study, Back Home, Other and Non-recurrent trips are naturally identified using mobile phone data. Other purposes can be inferred with much lower accuracy and in our view these are best segmented through data fusion with other sources. Moreover, it can be argued that the need for finer segmentation is partially driven by the need to develop different demand models by purpose to obtain better trip matrices.
If the aim is to develop tour or activity based models it is best to request information on tours, that is the sequence of these stays, activities and trips. This is an area only recently explored by modellers but one that is likely to be particularly rich in insights. Nommon-Kineo has already produced tours and activity diaries for a synthetic population in an Activity Based Model.
An additional type of user that may be important in some cases is the distinction between locals and “non-residents” or roamers. This can normally be obtained from mobile phone data identifying the country of residence, the length of stay, locations visited, etc.
Time of day
It is possible to specify a range of time of day slots but one should bear in mind two features of mobile phone data. First, the exact time of starting and ending an activity is only statistically approximated therefore very short time slots are currently not recommended for strategic modelling. Second, the user must specify what part of the trip should be in the time slot and the core study area: the beginning, the end or its middle. Mobile phone trip matrices are, in this sense, different from those obtained through intercept roadside interviews in the study area where this question does not arise.
Day of week/month
Normally, a month of data will be used to generate trip matrices or tour sequences for particular days or combinations of days. Therefore, the user may specify the month (or months) required and the type of days of interest: average working day, average Tuesday to Thursday day, specific day, etc. The greater the number of these days and time slots the more extensive the processing and cost of the resulting matrices. It is important to focus on what is really going to be used in practice.
Modes of travel
Modes of travel are difficult to distinguish from mobile phone data alone in dense urban areas and would need data fusion to be more accurately generated. The provision of modes of inter-urban travel is easier but still distinguishing cars from coaches may require additional data. Nommon-Kineo has developed approaches to estimate freight movements inferred from patterns of usage but this still benefits from classified counts where available.
The normal output will be person trips some of which may need converting into vehicle trips using traffic counts and/or occupancy rates obtained from other sources. Data fusion is the key for more accurate mode of travel identification. This type of task must be discussed with the data analytics company before specifying.
Select the right supplier
As mentioned above, there are two types of suppliers of mobile phone trip matrices: Mobile Network Operators like Telefónica, EE and O2 (perhaps with some adaptation by consultants) and Data Analytics companies processing MNO raw data and delivering trip matrices directly to end-users. This category includes Nommon-Kineo, Citi Logik and Teralytics, plus AirSage and StreetLight in the US.
The suggestions about choosing the right supplier of mobile phone insights that follow are obviously influenced by our own experience at Nommon-Kineo and, for that reason, should not be assumed to be entirely independent.
We believe it is most important to select a company that understands how the outputs from mobile phone data, trip matrices, tours or sequence of activities, will be used in practice, mostly through Transport Models. Domain knowledge is paramount not only in transportation but also for other applications of mobile phone data: Tourism, Geomarketing, Location Intelligence, Event Management and quasi-real time forecasting.
Ideally, this company should be able to process the raw mobile phone data with their own refined algorithms and analytics focused on how the end-user will deploy the trip matrices in models and apply them for planning and forecasting; we believe this is superior to obtaining a pre-processed standard product and then adapting to final use. Access to the raw mobile phone data requires an agreement with, and the trust of, the MNO that will naturally seek to protect the privacy of individuals through ironclad data protection arrangements. Reaching these agreements takes time.
It is also desirable to employ a company willing to discuss results and improve them if they are unsatisfactory to you. If the company is also willing to help you calibrate and validate your models using this new type of data this should be considered a positive bonus.
Overall, a good and realistic specification of the trip matrices required should go a long way to reduce, and even eliminate, any problems with the final product. Assistance with implementing and refining these trip matrices in a model should help an efficient use of this new data source.